DeepSeek: How Large Language Models Are Reshaping Technology and What Lies Ahead
In the past few years, artificial intelligence (AI) has transitioned from a futuristic concept to a transformative force reshaping industries, economies, and daily life. What does the future portend?
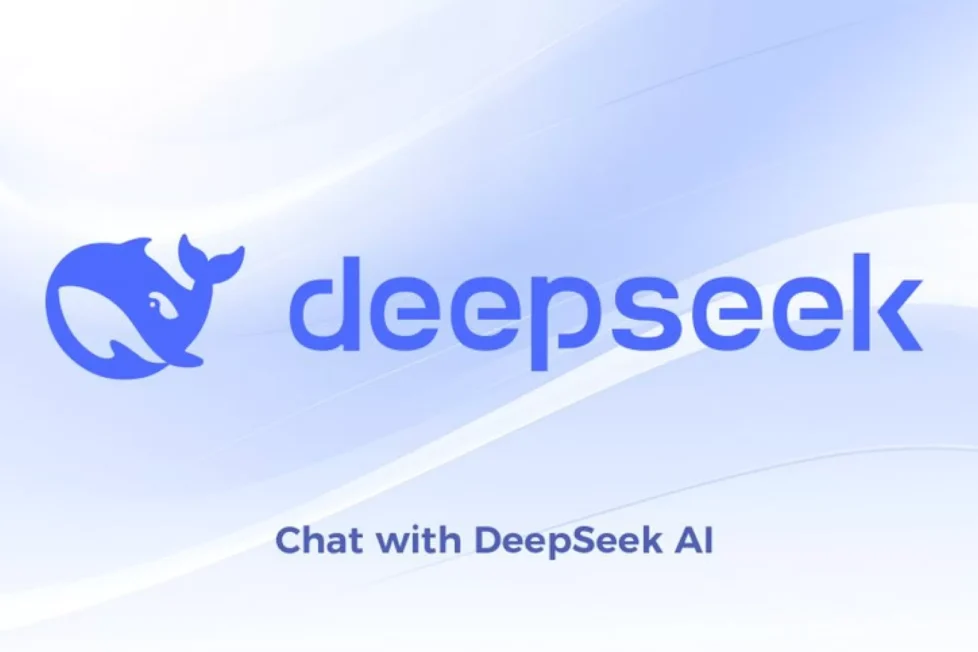